QAOA MaxClique#
Problem description#
Given a Graph
Classical cost function#
- create_max_clique_cl_cost_function(G)[source]#
Creates the classical cost function for an instance of the maximum clique problem for a given graph
G
.- Parameters:
- Gnx.Graph
The Graph for the problem instance.
- Returns:
- cl_cost_functionfunction
The classical cost function for the problem instance, which takes a dictionary of measurement results as input.
MaxClique problem#
- max_clique_problem(G)[source]#
Creates a QAOA problem instance with appropriate phase separator, mixer, and classical cost function.
- Parameters:
- Gnx.Graph
The graph for the problem instance.
- Returns:
- QAOAProblem
A QAOA problem instance for MaxClique for a given graph
G
.
Example implementation#
from qrisp import QuantumVariable
from qrisp.qaoa import QAOAProblem, RZ_mixer, create_max_indep_set_cl_cost_function, create_max_indep_set_mixer, max_indep_set_init_function
import networkx as nx
G = nx.erdos_renyi_graph(9, 0.7, seed = 133)
G_complement = nx.complement(G)
qarg = QuantumVariable(G.number_of_nodes())
qaoa_max_clique = QAOAProblem(cost_operator=RZ_mixer,
mixer=create_max_indep_set_mixer(G_complement),
cl_cost_function=create_max_indep_set_cl_cost_function(G_complement),
init_function=max_indep_set_init_function)
results = qaoa_max_clique.run(qarg=qarg, depth=5)
That’s it! In the following, we print the 5 most likely solutions together with their cost values.
cl_cost = create_max_indep_set_cl_cost_function(G_complement)
print("5 most likely solutions")
max_five = sorted(results.items(), key=lambda item: item[1], reverse=True)[:5]
for res, prob in max_five:
print([index for index, value in enumerate(res) if value == '1'], prob, cl_cost({res : 1}))
Finally, we visualize the most likely solution.
most_likely = [index for index, value in enumerate(max_five[0][0]) if value == '1']
nx.draw(G, with_labels = True,
node_color=['#FFCCCB' if node in most_likely else '#ADD8E6' for node in G.nodes()],
edge_color=['#FFCCCB' if edge[0] in most_likely and edge[1] in most_likely else '#D3D3D3' for edge in G.edges()])
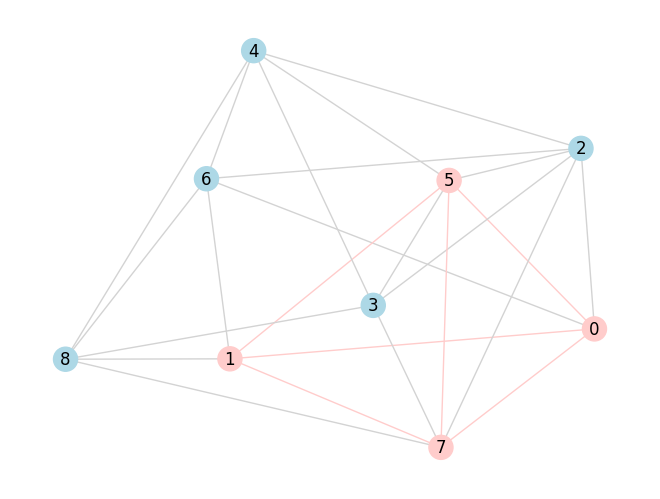